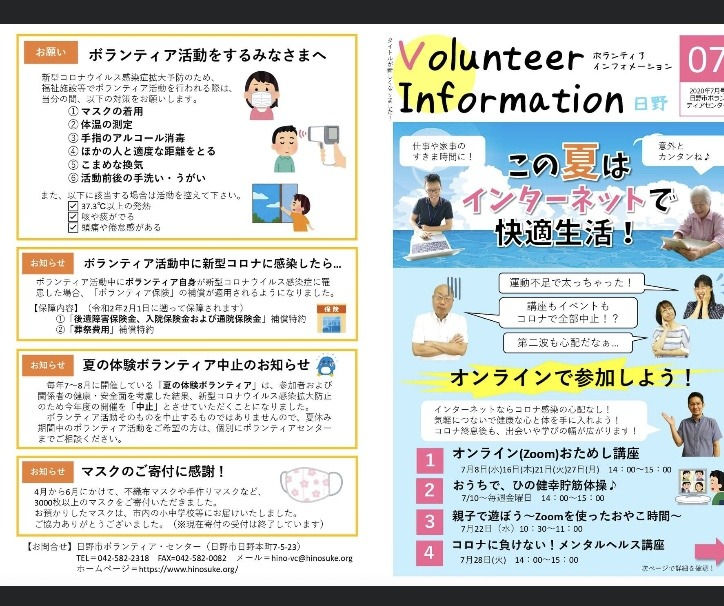
"""
pip3 install -U kaggle
# Tutorial:
# 1. Create an ML training task on kaggle.
# 2. Create a Kaggle notebook
# 3. Use Python, Pandas, Tensorflow.js and kaggle to create some models
# 4. Test the models
# 5. Implement your notebook on kaggle.
# 6. Submit the notebook to kaggle
# 7. View the results
# 8. Run the notebook on kaggle
# 9. Check if you can run the notebook on kaggle.
# 10. Submit your notebook to kaggle.
# No attached data sources
import pandas as pd
import kaggle
import sys
# Create the task
kaggle.Kaggle().create_task(
"UCI Dataset Classification",
"")
# Create notebook and notebooks
# Create a notebook
kaggle.Kaggle().create_notebook(
'')
# Create a local dataset
# Have to first copy the data to your computer
import os
filename = 'datasets/ucigames.csv'
os.system("cp datasets/{0}".format(filename))
# Do the data cleaning
data = pd.read_csv("datasets/ucigames.csv")
data = data.dropna()
data = data.head()
print(data.head(10))
# Create the predictors
predictors = [
"age"
"sex"
"race"
"class"
"classif"
"player"
"round"
"team"
"turnover"
"foul"
"fooball"
]
# Create a label
label = ["deaths", "golds"]
# Create the
Related links:
Comments